- TheTechOasis
- Posts
- Apple Outclasses ChatGPT & Top Predictions for 2024
Apple Outclasses ChatGPT & Top Predictions for 2024
š TheTechOasis š
Breaking down the most advanced AI systems in the world to prepare you for your future.
5-minute weekly reads.
TLDR:
AI Research of the Week: Apple Outclasses GPT-4V
Leaders: My Top Predictions for 2024
š AI Research of the week š
First and foremost, Happy New Year!!
Out of all AI news at the end of 2023, the fact that Apple is finally putting itself out there is probably the most important of all.
Although released back in October, an Apple model named Ferret has been making headlines recently.
Not only is it a first of its kind, but also beats ChatGPT-Vision in several tasks while offering new cues to what Apple may be cooking for the world in 2024.
The Importance of Getting Things Right
Out of all the issues you can face with a Language Model, hallucinations usually come first.
As Large Language Models (LLMs) are essentially stochastic word predictors, there is always some certain level of unpredictability in every response they give.
Consequently, when using these models, you must provide the model with relevant context in the actual prompt so that its response isnāt made up.
For instance, if you are preparing a summary of a news event that took place recently and, thus, the model does not know about it, you embed the article directly into the prompt so that the model uses it.
This process is called āin-context learningā, providing the relevant context for the response in real time.
This works because LLMs have seen so much data during training that they are capable of using data they have never seen before effectively, a process we describe as āzero-shot learningā or āfew-shot learningā when you provide many examples.
However, while grounding the model on text data is as simple as appending the required data into the prompt, grounding the model on images is a different story.
In other words, what if the necessary ācuesā the model needs to answer effectively are objects in an image?
For example, semantically speaking, the response to the question āWhat can I cook with thisā while pointing at a frying pan will be different depending on the ingredients in the table.

As context matters, grounding is essential, yet extremely complex to achieve in multimodal situations.
Adding insult to injury, when pointing to objects in an image to help the MLLM perform better, objects most often have irregular shapes, are partially covered by other objects, or can be poorly visible due to resolution issues.
This makes MLLMs extremely hallucination-prone. But Appleās Ferret MLLM has a solution for this.
Free-form Referring and Grounding
Put simply, Ferret is the first MLLM that successfully performs referring and grounding on any object in an image in a free-form style.
In other words, it can successfully refer to any object in an image or ground its responses on any object in that image.
All in one model.

As we can see in the image above, the user can point to objects in an image, like the dog, and the model correctly identifies it as a Corgi, establishing spatial relationships between objects, or even performing adversarial predicting.
But GPT-4V can do these things, right?
Yes, but ironically performs worse in many of such instances, despite Ferret being hundreds of times smaller.
But how is this possible?
The key is what Apple researchers define as hybrid representations. It sounds complicated, but itās not.
When you give an image to a standard Transformer-based computer vision model, it performs what we call the attention mechanism.
Itās a fairly similar process to what ChatGPT does to process a sequence of text, but applying special patterns to account for the structure of the image.
However, unlike humans, which process images pretty intelligently, todayās machines process images by brute force by applying global attention.
For instance, if you look at the image in this link, it has 1 billion pixels. This allows you to see even the most intricate details in buildings hundreds of meters away from the source.
But how long did it take you to figure out which city was that?
As humans apply attention both globally and locally, as soon as you recognize buildings like the Empire State, you know that is New York, and you donāt have to look anywhere else in the picture from that point onwards.
In other words, instead of scrutinizing the billion pixels, you just required a handful of them to acknowledge the place.
Modern frontier models, however, will systematically analyze the whole image, which, considering itās a billion pixels big, would take huge computational resources and a considerable waste of time.
But while Ferret applies a global attention mechanism, it also includes a free-form localized component that makes it truly unique.
That is, the key behind Ferretās success is what they define as a spatial-aware visual sampler.

To comprehend what is that, we need to take a look at what Ferret takes in as input:
An image
The text prompt
A visual cue in the form of a pointer, a box, or a free-form shape (like a catās tail)
First, as with any default MLLM, an image encoder processes the image to capture its semantics and an embedding lookup does the same for text.
But here is where things get interesting.
Ferret takes the output of the image encoder (the representation of the image) and, using a binary mask, identifies the pixels in the image that fall inside the visual cue.
A binary mask simply puts a ā1ā over pixels inside the selected region and ā0ā on the rest.
Next, it inserts both inputs into the spatial-aware visual sampler, which in turn follows a three-step process:
It first samples a discrete number of points inside the region
Then, using K-neighbors, it identifies proximate points and fuses their features into one
Finally, for each set of points, it performs a pooling, aggregating each set into one unique feature that encapsulates the coordinates of the object with the representation of the object, hence the name āhybridā
But what does this all mean?
In laymanās terms, once you indicate the region of study, it performs an additional attention process focused on that area.
In other words, while standard multimodal models will have a unique representation of the whole image, Ferret also has an updated, detailed representation of a certain part of the image.
Additionally, as the original pixel features already include the information about the global attention process done earlier, the model is not only capable of paying close attention to the object in the region, but it also considers the semantics of its surroundings, which in turn allows the model to understand that object in the context of the whole image.
This whole process allows Ferret to have unique capabilities that not even models like ChatGPT have, like free-form selection and, above all, output grounding.
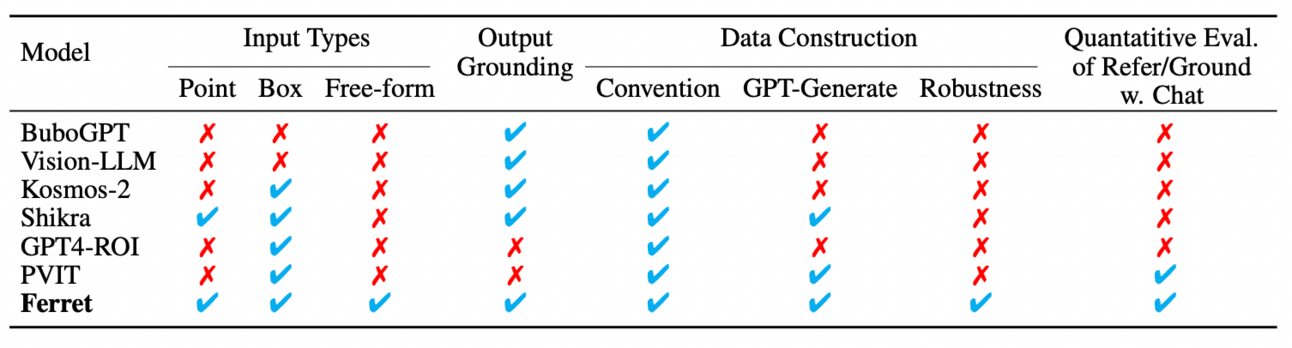
But what do we mean by output grounding?
Put simply, the model can use the context of the image in its response, all the while having considerably fewer hallucinations than other state-of-the-art models like GPT-4V.

Very impressive results you can also check in the original paper, but what cues does this give to us about Appleās strategy?
Small is Better
With models like Ferret and breakthroughs like last weekās Flash LLMs, itās clear that Apple has no interest in playing the big model game that Microsoft or Google seem to be all about.
Frankly, it makes total sense, as Appleās main selling points are consumer-end products that, no matter the innovation, will require tricks and tips to run those models, if ever possible.
But Appleās impressive results signal that changes are coming to their products pretty soon, by leveraging Ferret-style models for:
More context-aware image and video editing
Better Siri interactions
An improved health offering to support users with questions about their physical wellbeing
And much more, because an improved referring and grounding system like Ferret is essential to making MLLMs production-ready.
Is this Appleās Year?
Historically, Apple has always been great at not being first in a technological race but eventually taking the victory.
The smartphone, the digital watch, headphones⦠none of those markets were started by Apple, but everybody knows who eventually won.
Will AI be the same?
š«” Key contributions š«”
Ferret introduces the concept of spatial-aware visual samplers, a component that allows the model to identify key objects in an image and refer or ground its responses to them
It beats GPT-4V in many tasks despite being hundreds of times smaller
š¾ Best news of the week š¾
š CaliExpress, the first autonomous restaurant
š„ Leaders š„
What will happen in 2024?
2023 has been an amazing ride for AI.
We saw the arrival of all types of general-purpose language models that are capable of executing a plethora of tasks and can be combined with other components to give them perception capabilities and create multimodality.
But as Sam Altman stated a few months ago, ā2024 will make 2023ās AI seem quaint.ā
And today we are going to analyze what 2024 could look like along the lines of what new models may come into our lives, enterprise-grade AIs, regulatory scrutiny, and⦠whether AGI will likely happen in 2024 or not.
The Year of Efficiency
As claimed last week, although I do expect new more powerful models, I do expect that much of the innovation will be driven by the desire to improve the quality/size ratio.
That is, proof has been accumulating over the last months that we can get incredibly good models at very small sizes: Fuyu from Adept, Orca, and Phi from Microsoft, Ferret from Apple (look above), and many more.
These models often compete in many regards with the big guys despite being at least two orders of magnitude smaller.
Mixtral 8Ć7B from Mistral is already only 13% far from GPT-4 at MMLU, a popular multimodal benchmark.
And although I do expect proprietary models to continue to lead the race, I predict open-source models getting to the GPT-4 level during 2024, which could cause a dramatic shift in enterprise GenAI toward open-source models.
Indeed, many of these models have been trained with synthetic data generated by the likes of GPT-4 and using training techniques that essentially teach these models to imitate the big guy, a process called distillation.
But for most cases, enterprise or not, you donāt need the best model in town to obtain great results, so the trade-off between costs and performance will no longer be logical for companies using proprietary models.
Model-wise, this is a list of models coming in 2024 that you should be paying attention to, like:
LLaMa 3 family
Adeptās ACT-1
Gemini Ultra (already announced), by Google
A 100-billion-plus proprietary model by Mistral (or larger)
GPT-5 by OpenAI
OpenAIās Q* and Googleās equivalent
Appleās Ajax model
Modality-wise, I also expect Multimodal models to start generating consistently good video data besides images and text.
But, if one thing is clear today, is that AI at this point is still uncharted territory for most companies. A lot of hype around it, but little to account for in the real world.
I predict that will change in 2024. Hereās why.
AI Everywhere, Unlessā¦
As we discussed, next year wonāt be about the next leap in terms of capabilities for AIāunless Q* changes everythingābut a considerable improvement in model quality, safety, accuracy, and overall performance.
And if thatās true, it means that companies around the world are going to start to throw AI stuff and projects into the wall until something sticks. In other words, I expect AI investment to increase exponentially.
In that regard, although a considerable part of that investment will involve computing and model deployment, I also think companies will invest heavily in strategy and governance.
Roles like the Chief AI Officer, something the US federal government is already enacting in its different government agencies, will become a common theme in the corporate world.
Also, AI-specialized teams in prompt engineering, RAG deployment, and such will be more in demand than ever.
But a great part of all this progress will depend on tackling the elephant in the room, regulation, which is far from being a clear-cut topic.
First and foremost, there are considerable differences in the way the US and the EU/UK are approaching this concern.
In the case of the horse leading the charge, the EU, their approach is heavily focused on regulation per use case, meaning that the appropriatenessāand even the legalityāof AI will depend on āwhat is going to be used forā.
Also, they put a lot of emphasis on the responsibility and safety part of AI; explainability, transparency, and robustness are critical to its principles.
The UK follows a similar stance, with their approach underpinned by 5 principles: Safety, security and robustness, appropriate transparency and explainability, and fairness.
On the other hand, the US, at least from the looks of Bidenās recent Executive Order, although they also follow the same oratory, is much more focused on foundation models, restraining training thresholds, and the fear of unexpected emergent capabilities.
In my view, the USā EO is much more heavily influenced by big tech lobbyists, as it seems that they want to turn the AI industry into something similar to the drug industry. Thus, the possibility of seeing a āFederal Drug Administration for AIā looks reasonable.
This EO and its hawkishness regarding training foundation models is nothing but positive for private big players and a clear attack on open-source, as explained by Andrew Ng.
Personally, I think that regulators should worry less about models in themselves and more about how they are going to tackle the topic of alignment.
However, the big guys are still far from being safe, as even though the regulatory scene is unclear, class actions are going to rain on these companies by having trained their models using data they had no business in using.
And some of them are askingāand could succeedāfor the destruction of ChatGPT.

Subscribe to Leaders to read the rest.
Become a paying subscriber of Leaders to get access to this post and other subscriber-only content.
Already a paying subscriber? Sign In