- TheTechOasis
- Posts
- Microsoft Spyware, Google's Massive Bet, & KAN networks
Microsoft Spyware, Google's Massive Bet, & KAN networks
🏝 TheTechOasis 🏝
part of the:

Welcome to the newsletter that keeps you updated on the latest developments at the cutting edge of AI by breaking down the most advanced systems in the world & the hottest news in the industry.
10-minute weekly reads.
💎 Big Announcement 💎
Next week, I am officially launching my community, TheWhiteBox, a place for high-quality, highly-curated AI content without unnecessary hype or ads, across research, models, markets, and AI products.
With TheWhiteBox, we guarantee you won’t need anything else.
No credit card information required to join the waitlist.
🚨 Week’s Update 🚨
Hello again! This week, we have news from Microsoft, Anthropic, OpenAI, contortionist robots, and more.
Starting with Microsoft, they presented their new AI laptops. Besides being the self-proclaimed most powerful laptop, even superior to the Macbook M3, they will include an on-device LLM, Phi-3-Silica.
The most controversial announcement was the feature ‘Recall.’ Phi-3-Silica will monitor every action you perform on your laptop, allowing you to perform a semantic search of everything you’ve done. This video shows a clear example.
This superpowerful feature has been met with huge controversy, calling it “spyware”, but some pointed out it would become a cleaer easy path to our private lives. Even Elon Musk reacted negatively.
Moving on, Anthropic made a huge announcement with its efforts in demystifying LLMs. They studied how different combinations of neurons in Claude 3 Sonnet elicited very specific behavior.
More research on this could one day lead to the capacity to block certain behaviors of models to make them safer to use while giving us a deeper understanding.
We also saw OpenAI’s worst week in a while, with two prominent researchers, co-founder Ilya Sutskever and Jan Leike, leaving. The latter even claimed OpenAI no longer prioritized safety, which is a very concerning statement. I cover all that happened in my Medium blog post.
Returning to Microsoft, CTO Kevin Scott announced how big the company was betting on AI. Specifically, they add around 72,000 NVIDIA H100s, the state-of-the-art AI hardware, every month, a $3 billion-a-month investment.
For reference, Mistral is reported to having just 1,500 H100s in total. This just goes to show how unbalanced AI is becoming.
To end on a positive note, Unitree has presented a $16.000 contortionist robot that can perform various actions and even fold itself for packing.
We might be entering the age of “affordable“ robots, an age where everyone gets their robotic friend to help on chores or cook.
💎 Sponsored content 💎
Your Everywhere and All-in-One AI Assistant
Imagine an AI companion that works across any website or app, helping you write better, read faster, and remember information. No more copying and pasting—everything is just one click away. Meet Flot AI!(Available on Windows and macOS)
🧐 You Should Pay Attention to 🧐
The Biggest Pivot in History
KANs & The Challenge on Deep Learning
😦 The Biggest Pivot in History 😦
Heavily speculated for months, Google has finally revealed its plans regarding AI and the future of search in what might become one of the most brutal business model pivots in capitalism's history.
But does this massive bet of unknown consequences imply it’s time to short Google?
In my humble opinion, Google appears stronger than ever. And the reason is even less obvious:
Proteins and diversification.
Adapting to the Changing Times
For years, the death of search has been proclaimed. While the premise was correct, people were too skeptical about Google’s capacity to overcome this issue.
The reason for this was that search represented upwards of 58% of their direct business at the time while also being heavily related to an additional 20% derived from ad shares from YouTube and the Google Network (display and on-site ads, respectively):
Simply put, to survive, Google had to disrupt (euphemism for ‘kill’) its main revenue source, which seemed complicated. Throughout 2023, things only got worse, but with the release of Gemini, things changed.
Ready to Fight Anyone
Although many of us probably got too carried away by Google's bad news and poor timing execution, if you look carefully, they are brilliantly positioned.
If we look back, as with any for-profit model, the pressure to pleasure its shareholders eventually lured Google into giving more and more presence to companies willing to pay to be on top, creating the perfect storm.
On the one hand, searches were filled with keyword-optimized blogs that offered no value, making the search process hideous and cumbersome.
Over time, the first three or four of the 10 blue links became sponsored links, another annoying experience for the user.
But in last week’s Google I/O conference, the company finally took the step we were all (except incumbents) hoping for: fully embracing AI-based search.
Changing the game
Long story short, Google has literally copied Perplexity’s playbook, turning the search process into a activity where humans do the asking and AIs (Gemini) do the clicking and generate an immediate response.
An Unanswered Question
At the risk of massive traffic drops in many websites, Liz Reid, head of search since March, claims AI will lead to more clicks on the open web.
Honestly, that’s so hard to believe. Sure, if your website is consistently picked up by Gemini to respond to the user’s request, you will increase traffic, but this is extremely hard to predict.
Gemini is a Large Language Model (LLM), a total black box. This means that the step where the model plans the search, aka chooses what websites to search, is highly unpredictable.
In layman’s terms, the process is non-deterministic, meaning that an exact, letter-by-letter search might yield slightly different plans in each case and, thus, slightly different website candidates.
Long story short, this means that even if they don’t want to affect your traffic, they can’t guarantee that it will.
However, I still feel Google is making the right moves.
An AI-centered Cash Cow
To begin with, it may seem that Google is using the Google Network (around 10–12% of current revenue, depending on the cited source), the product for on-site ads, as the sacrifice lamb. Furthermore, display ads (on YouTube or other Google products, which account for around 20% of revenues) don’t seem to be particularly affected by all this.
However, we must pay attention to the trend among big tech companies, which are all slowly becoming cloud providers.
In Google’s case, it represents 10% of the business and is growing fast. As demand for AI will continue, with a projected 28% Compound Annual Growth Rate until 2030, this revenue segment will continue to increase considerably over time, a huge win for Google’s strategy.
Nonetheless, Google is beyond prepared for that increase in compute, much more than its rivals actually:
However, the most exciting future revenue sources come from AI products: Gemini and AlphaFold.
An Everything Giant
If you watch carefully, Google plays a role in every single layer of the AI pipeline:
It builds its own AI hardware, TPUs, meaning it has less dependency on hardware providers like NVIDIA (still a customer, though)
It offers infrastructure services through its cloud computing business
It builds and serves its proprietary models, the Gemini family
It will become omnipresent at the application layer, as it has the influence and the cash to verticalize into any industry it wishes.
Delving into the last bullet point, we see this already in healthcare, with Med-Gemini as a clear example.
However, the brightest opportunity in Google’s healthcare strategy materialized recently, with Isomorphic Labs, to monetize their industry-changing discoveries with AlphaFold 3.
Alphafold is a protein folding predictor. In other words, it inputs a sequence of amino acids and outputs their protein structure.
Based solely on previous versions of the model, it is already considered a vital tool for biologists to fuel drug discovery. According to investor and billionaire Chamath Palihapitiya, it could easily be a $100 billion business alone.

What We Think
For over a year, Google finally seemed vulnerable due to its excessive dependency on search. Of course, this new diversification showcases risks galore (moonshots in healthcare, strong competition in AI, and the uncertainty of this new search paradigm).
But with the advent of Gemini and the amazing discoveries in Google Deepmind in biology and robotics, added to Google’s insane compute capabilities, which will be essential to delivering AI at the intended scale, suggests that Google might be hitting all the right notes and might be primed to become what many desired for years: a well-diversified multiple trillion dollar company.
🔍 KAN Networks, A Revolution Coming to AI? 🔎
Few papers released this year have spurred more controversy than the presentation of Kolmogorov-Arnold Networks, or KANs, a new type of neural network that could potentially substitute one of the main pillars of the AI revolution and essential components of tools ranging from ChatGPT to Stable Diffusion, or Sora.
This may sound like a handful of overly pompous claims, but as you'll see right now, this is exactly what we are looking at.
Why Does AI Work?
We first need to understand the main building blocks of current frontier AI models to understand the huge impact of KAN networks.
Although I really recommend reading my blog post as an introduction to AI, a few elements of AI are as influential today as deep learning (DL).
Simply put, today, nothing creates more value (at least in private and open markets) than Deep Learning: ChatGPT, AlphaFold, Sora, AlphaGo…, and the list goes on.
But what is Deep Learning?
In short, DL involves using neural networks to find latent relationships in data, from using house parameters to predict its price to predicting the next word in a sequence, like ChatGPT:

But why do neural networks work?
Theoretically speaking, they are based on the Universal Approximation Theorem, which states “that a neural network with at least one hidden layer can approximate any continuous function to arbitrary precision with enough hidden units.”
But what does that mean? Let’s see an example.
Below is a standard depiction of a shallow (1-layer) multi-layer perception (MLP), in which the relationship between the inputs and the outputs (the black box we just showed) is defined by weighted linear combinations of hidden units, usually known as ‘neurons.’

Source
In particular, each of the ‘hidden units’ depicted as ‘h1’ to ‘h5’, perform the calculation below:
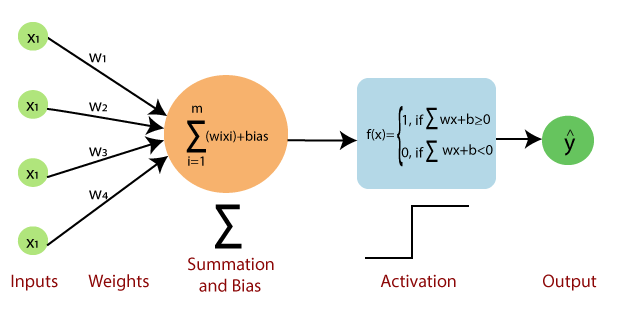
Broadly speaking, each neuron is a linear combination of the inputs in the previous layer (in this case, the inputs to the model), which are weighted by parameters w1-w4 and the neuron’s bias.
These ‘weights’ are what the model adapts during training to learn to perform the task.
Then, the entire calculation is driven through an activation function, a non-linear function that determines whether the neuron activates or not, hence why they are called neurons.

The ReLU activation function
For example, for each neuron that uses this function above, the neuron fires if the result is positive; if the result is negative, the neuron doesn’t. Long story short, we can find very complex relationships in data with enough of these neurons.
If you want to truly visualize why these combinations of neurons work, including deep neural networks, I highly recommend you look at my recent blog post addressing why standard neural networks work.
But now, suddenly, a group of researchers from universities like MIT or Caltech say there’s a better way. And that way is Kolmorogov-Arnold Networks.
Are we ready to ditch the very foundations that have taken us so far?
KANs, A New Start?
As you will see in a minute, I was not overstating the impact that KAN networks can have on the future of AI.
Despite their ubiquitousness, MLPs are a pain in the butt. This becomes apparent just by looking at Large Language Models (LLMs). As you can see below, MLPs play a crucial role, as Transformers are the building blocks of LLMs:

However, according to research by Meta, 98% of the model’s FLOPs (the amount of operations the GPUs perform to run the model) come only from these layers, with just 2% coming from other critical pieces like the attention mechanism, the core operator behind LLMs’ success.
Therefore, while the Universal Approximation Thereom promises that you will eventually approximate the mapping you desire, it doesn’t specify how many neurons you will need, which usually equals billions for very complex cases like ChatGPT.
So, what if there was a better way?
Alternatively, KANs follow the Kolmogorov-Arnold Representation Theorem (KART), which states “that a summation of univariate functions can find the mapping to a multivariate function with arbitrary precision.”
In other words, while MLPs learn the weights on the network's edges, KANs put learnable activation functions, represented as splines, in the edges, and the nodes are simply a summation of these splines, following the KAR theorem.

Shallow (one-layer) representation of both methods (MLPs left, KANs right)
I know that sounded like gibberish, but I promise it will all make sense in a minute.
If we recall the structure of MLPs earlier discussed, each neuron is a combination of learnable weights, represented as the connections to that neuron, with a fixed activation neuron.
Consequently, while weights are located at the edges, the nodes are the actual neurons, where a fixed activation function decides whether that node fires.
With KANs, the idea is to find the set of one-dimensional functions that, added, will approximate the relationship we are looking for.
However, we can make the explanation even more intuitive. Let’s take a look at the example below:
Let’s say we want to train a neural network to approximate the equation exp(sin(pi*x)+ y²), which takes in ‘x’ and ‘y’ as inputs and generates an output.
Thus, the process is:
Squaring ‘y’
calculating the sine function of the product ‘π * x’
And applying the exponential function to the summation of steps 1 and 2
Fascinatingly, after training, the KAN network indeed finds the set of splines that mimics the exact function:
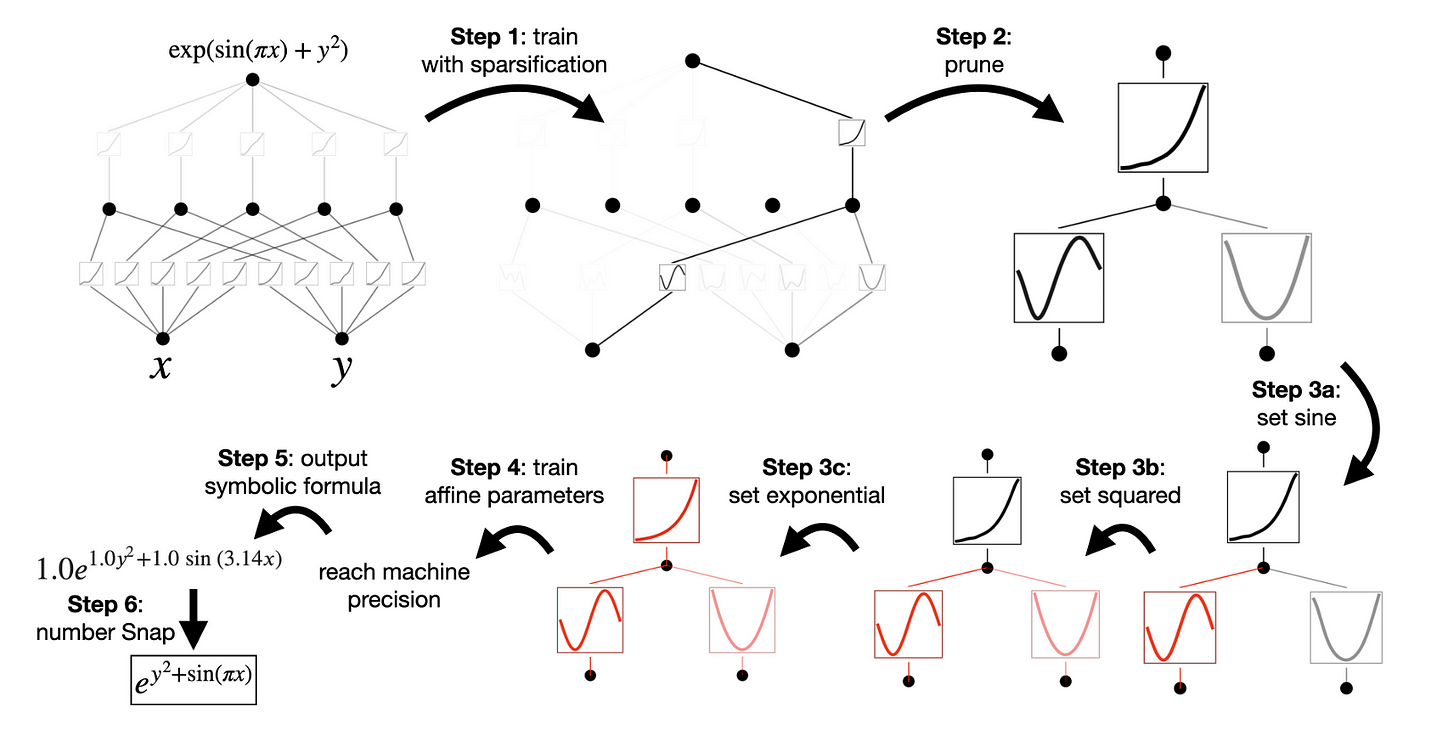
Specifically, the KAN network becomes a much simpler representation of three splines that, similarly, take the following shapes:
a square function applied to ‘y’,
a sine function with a frequency proportional to the constant π, applied to x.
As nodes are sums, the results are added and finally used as input to the exponential function, giving the same final result.
Of course, this is a toy example because we already know the symbolic equation that rules this input/output relationship.
But what if we didn’t know the function? That’s precisely the point of AI models like ChatGPT, and the reason why neural networks have become so popular as a tool for discovery.
In 2022, a group of researchers rediscovered Newton’s gravity laws with a neural network. They fed it the observations (when ‘x’ happens, ‘y’ happens) based on known solar system dynamics, and the model autonomously ‘discovered’ the laws.
Crucially, the power of neural networks to uncover nuanced patterns in data allowed it to discover gravity despite not being told crucial attributes like planet mass (it discovered those, too).
That’s why neural networks are so valuable; they stumble upon things that humans can’t and eventually adapt to find an approximation to the function (the equation) that governs that mapping.
But we still haven’t answered the question: Why KANs over MLPs?
Interpretability and speed
As seen above, KANs are beautifully interpretable (at least at lower dimensions).
But they are also much, much cheaper to train, as they require much fewer parameters than a standard MLP for the same task.
For those reasons and their clear expressiveness, KANs are spurring quite a hot debate, with overly enthusiastic claims that ‘Deep Learning as we know it is dead.’
But is it?

What We Think
Hold your horses for one second, as some disclaimers need to be pointed out.
KANs are unproven at scale. We don’t know whether the clear advantages they have shown with small trainings still apply to large-scale training pipelines like the ones we are growing accustomed to.
They haven’t been tested in sequence-to-sequence models such as LLMs. We don’t know if KANs work to model language.
They are not adapted for GPUs. Researchers specifically mentioned they committed to theoretical and low-scale proof that KANs are better than MLPs in some key cases, but not to prove their efficiency with our hardware. Current Deep Learning architectures like the Transformer have been basically designed specifically for GPUs. If KANs can’t achieve such efficiencies, they aren’t going to be used, period.
KANs are also much slower to train and run. That being said, new implementations like FastKAN are appearing, increasing the inference speed by more than three times.
But if they deliver on their promise, the entire world of AI will be thrown into chaos, just like our world when we realized that the Earth was not at the center of the universe.
If something with three-order-of-magnitude cost decrease potential comes up, companies behind the insane investments in GPUs we are witnessing might reconsider their position if AI suddenly becomes simpler and cheaper to create.
We don’t know the answers yet, but the excitement is hard to hide.
🧐 Closing Thoughts 🧐
This week has been more about the controversies than pushing the frontier of AI. We are seeing how the corporations driving the industry, like Microsoft, are desperately trying to shove the technology down our throats to justify their insane valuations, and OpenAI is suffering some serious PR setbacks.
Yet, to this day, the expectations have been largely unmet. Since the AI explosion, AI companies have seen a $2.5 trillion increase in valuation. However, their explicit AI revenues have accounted for a pathetic $20 billion, 120 times less.
Unless AI starts to deliver, this is the biggest bubble in the history of capitalism, no question.
Give a Rating to Today's Newsletter |
Do you have any feelings, questions, or intuitions you want to share with me? Reach me at [email protected]